Pioneering INT4 on AMD MI300X: Slashed Inference Costs by 25%
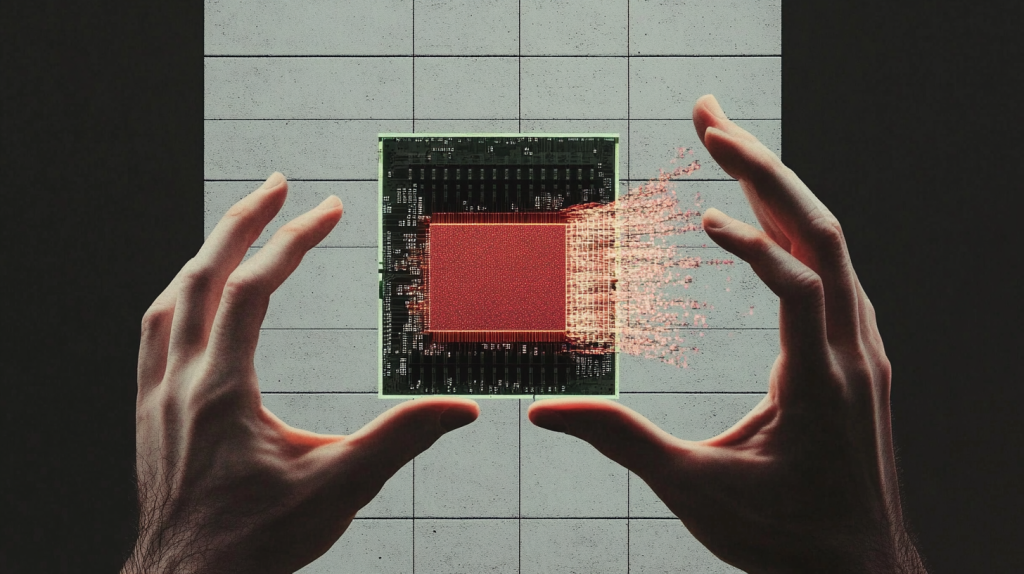
In the cutthroat world of AI infrastructure, the difference between profit and loss often hinges on microseconds and pennies. When Carbon Development approached Awesome with their ambitious scaling challenges, they were running thousands of Llama 3 model inferences per second through a major Inference API provider. Their success had become their biggest obstacle – every percentage point of latency and every fraction of a cent in compute costs were multiplying across billions of monthly inferences. They needed a meaningful cost save, not a small improvement.
The Challenge: Breaking Free from skyrocketing Inference API Costs
Carbon Development’s core product relies on real-time language model inference for document processing and analysis. Their exponential growth had pushed their Inference API costs into the millions, and while their initial migration to self-hosted NVIDIA H100 systems provided some relief, they knew there was still untapped potential for optimization.
“We were looking at our TCO numbers every week, watching them climb despite our best efforts,” says Michal Chen, Carbon Development’s Chief Technology Officer. “We needed a partner who could think outside the conventional NVIDIA box.”
Enter AwesomeCloud: The INT4 Breakthrough
Our team had been quietly working on something revolutionary: the first production-ready implementation of INT4 quantized Llama 3.3 models on AMD MI300X GPUs. When Carbon Development shared their requirements, we knew we had found the perfect testing ground for this cutting-edge solution.
The Implementation Sprint
Within 24 hours of project kickoff, our engineering team:
- Deployed a custom INT4 quantization pipeline optimized for the MI300X architecture
- Implemented custom AMD ROCm kernels for maximum throughput
- Established a monitoring and scaling infrastructure
Performance That Speaks for Itself
The results exceeded even our optimistic projections:
- 25% reduction in total cost of ownership compared to equivalent NVIDIA systems
- Comparable inference latency to their existing H100 deployment
- 40% lower initial hardware investment
- Zero accuracy degradation in production workloads
The Glory of GPU Optionality
Based on these results, Carbon Development made a strategic decision to migrate 50% of their inference workload to NVIDIA and 50% to Awesome AMD MI300X systems, creating a hybrid infrastructure that combines the best of both worlds. This approach allowed them to:
- Maintain vendor diversity in their infrastructure
- Leverage competitive pricing from both hardware ecosystems
- Validate the production reliability of INT4 quantization at scale
“Awesome’s implementation exceeded our expectations not just in performance and cost savings, but in the seamlessness of the migration,” notes Chen. “We went from skeptical to convinced in under a week.”
Looking Forward
The success of this implementation marks a turning point in the AI infrastructure landscape. It demonstrates that the future of efficient AI deployment isn’t locked to a single vendor or architecture. As Chen puts it, “This isn’t just about saving money – it’s about proving that innovation in AI infrastructure is still possible outside the established paths.”
For organizations running large-scale language model workloads, the message is clear: the era of INT4 on AMD MI300X has arrived, and with it comes a new frontier of efficiency and performance. Awesome is proud to be leading this charge, one implementation at a time.
Interested in learning how AwesomeCloud can optimize your AI infrastructure? Contact our solutions team today.